Here’s how big data is used to fight crime
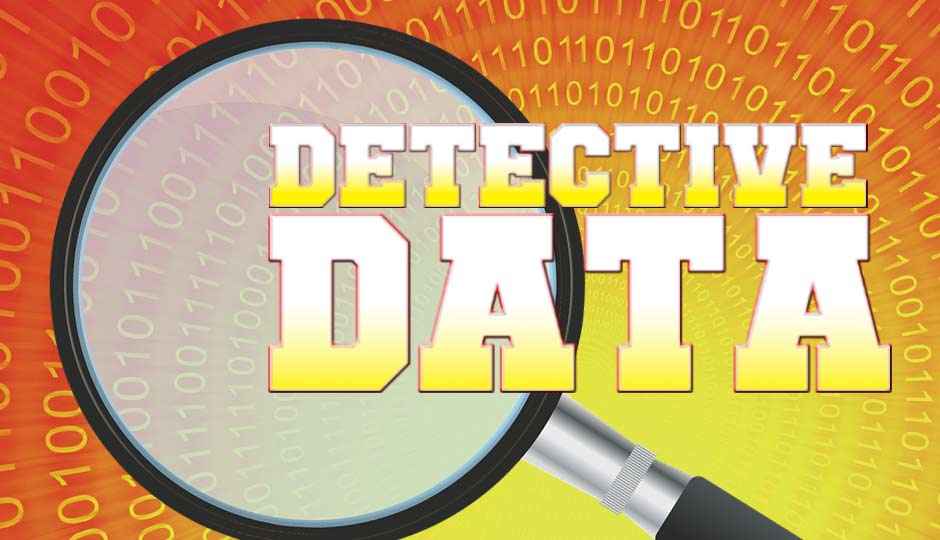
The long arm of the law just got a little longer thanks to Big Data. But are you willing to let go of your privacy to be safer?
Imagine walking down the street in the near future, the IoT has reached its peak and its ever pervasive nature entails that anything and everything in and around you is connected, and petabytes of data is being sent from trillions of connected systems to intensely powerful analytical systems. You are decked with gadgets – smartphone, smartband, and an AR visor are bare minimum.
As you continue walking, your AR (augmented reality) visor has your morning schedule listed and its navigation assistant directs you to take a turn to save a few minutes. You turn into the corner and your visor camera analyses every object and every person in front of you, highlighting those whom you may be connected to on social media. Your visor immediately turns a shade of orange and outlines a man 150 meters ahead of you. Your visor camera hasn’t identified the person but the mere fact that he has been pacing to and fro rather hurriedly gets flagged. You notice that some of the CCTV cameras on the street turn in his direction.
Seconds later, the orange alert turns red and so does his outline. You become alert and come to a halt, the visor navigation directions reroute to show you the fastest way to safety. You notice that every other person on the street just received the same alert on their visors and they’ve all started moving away from the suspicious person. A counter soon pops up on your visor, the cops are on their way and they’ll reach in barely two minutes. The area you’re in has a heightened police density owing to the number of crime incidents here. You’ve double-backed to the earlier street corner, notice that the cops are already there and they’re already observing the person. A crime has not taken place so they can’t arrest anyone. Two officers from the other end of the street slowly walk towards the suspect with a smile on their face…
It might seem a little too futuristic, but similar systems have already been designed and multiple pilot programmes are being run in cities and towns across the globe. Predictive Crime Analytics is very much in the present thanks to Big Data.
Whoops! I just got flagged by the cops…
Predictive Crime Analytics
While not quite the way Minority Report – a Tom Cruise movie about predictive crime – pictured it, quite a few software already deal with Predictive Crime Analytics with even technology giants such as IBM and Hitachi coming out with their systems. Needless to say, quite a lot of questions have been raised regarding the efficacy of such systems. PCA is doing exactly what analysts and detectives have been doing for decades – poring over all the evidence to identify patterns and shortlist suspects. The only difference is that our methods of obtaining information have multiplied to the extent that humans will soon not be able to handle the enormous amount of data that needs to be analysed.
Enter, Machine Learning
Traditionally, databases would be populated manually by humans so there was a defined structure but this would also mean that the very same structure ended up being restrictions to the kind of data that could be stored in the database and thus a portion of the data would be discarded. As technology progressed, databases evolved to attain a state where the human element could be taken out of the situation. Now you can store all kinds of data and not worry about giving it structure. That comes later. With IoT gaining momentum, the sheer volume of data that’ll be produced calls for even quicker analysis of the data and with that comes Machine Learning algorithms that can sift through massive data sets to ascertain patterns or anomalies.
Machine learning is being used across a multitude of application scenarios. If you have a smartphone, then the very reason that Google Now, Siri or Cortana can easily understand voice commands with an Indian accent is because of Machine Learning. Millions of us have tried out these services and the more it gets to hear, the better it picks up. If you’re into writing blogs then you’ve obviously come across Google’s Adwords which can show you which keywords are the best for your topic and then rank keywords based on search volume. The more you feed any machine learning system, the more it learns and the faster it becomes. It’s only outlier cases that can throw off such systems and that too for a short while. So how does machine learning work? Here’s a quick primer:
Machine Learning requires you to feed a set of learning algorithms voluminous amount of data. This data is broken down into three parts. First comes the ‘training set’ which allows the ML algorithms to easily figure out how to look for patterns, which parameters to prioritise (or give the highest weights). This data set would constitute roughly 60% of the entire data. Then comes the ‘validation set’ which helps the ML algorithms fine tune the parameters of various patterns. This forms about 20% of the entire data and lastly, you have the ‘test set’. Given all the learning the system has done, you can figure out how accurate the system is to see if further training is required before opening the flood gates.
Predicting crime with Machine Learning
What kind of data?
Since we were talking about predicting crime, you can easily assume that criminal case files would be one of the first things to be fed to the system. Manual methods that have been used by analysts involved looking at variables like names of schools which were prone to have higher gang members and then cross-referencing that data with slang words on Twitter to see if anything was afoot. A human would assign weights to indicate importance of certain terms. For example, in India the word “Ghoda” literally translates to “Horse” but the erstwhile gangsters would use “Ghoda” to reference a pistol. So when a PCA system comes across such terms which seem a little offbeat for normal conversation then it gets flagged. And if this flagged incident seems to be centered around a physical location that’s known for violence then you’re looking at a higher probability of a crime being committed. The local law enforcement authority of the area can then be notified at which point a human can investigate. The system depends on validation so it will have to be notified if its ‘hunch’ was indeed on point. This is how latent Dirichlet allocation (LDA) functions in a PCA system.
And the data fed to such PCA systems would include:
- Biometrics – To recognise individuals.
- Body language patterns – To identify abnormal behavior
- Travel history – To see if Hot Zones are developing based on density of criminals
- Media consumption – To see if flagged content is being viewed
- Monetary asset levels – Lower levels of wealth would indicate a higher propensity to commit crime if the person has a criminal history
- Exposure to criminals – To see if they’re in bad company
- Feeds – To track movement of individuals
- License plate reader information – To track movement of individuals
- Environmental data – To track changes in Radiation levels etc.
And as more and more agencies adopt the same PCA system, the data sharing between individual systems would further enhance accuracy levels. And to increase the amount of data, all the above mentioned sources of data would be encouraged to hoard and provide even more historical data. Which brings us to the ever popular discussion on privacy. As we stand now, you can’t expect privacy for everything that occurs in the public domain. So will these systems obtain information from your private devices? Soon enough, yes. Or quite probably they already are. While national intelligence agencies of first world countries have been mining and generating metadata from your personal devices for a few years now, these PCA systems so far don’t seem to do so. Current systems rely on publicly available data but it won’t be long before you’re given a choice to voluntarily surrender your privacy to help such systems improve accuracy.
Are machines bigots?
One of the biggest arguments against law enforcement agencies is that the officers of the law tend to be biased towards a certain race or even community because historical data says so. So would feeding a PCA system the same data cause it to turn into a racist and bigoted system like what happened with Microsoft’s AI chatbot – Tay. Tay started off an experiment in conversation understanding and according to Microsoft, the more you chat with Tay, the smarter it becomes at making conversation. It’s a pity that Twitter went wild and started chatting with Tay using misogynistic and racist remarks. The AI soon picked up on this and assumed it to be part of a normal and casual conversation. And soon enough, it went from an innocent chatbot to a full-blown bigot that could out-nazi Hitler himself, all in under 24 hours.
So when you take historical data which says that members of a certain community have a greater propensity to commit crime, will an AI immediately flag him or her down? Not quite so. As the system learns more and more, it will make predictions and flag down individuals for officers to intercept, and the moment it receives feedback on whether the individual was indeed a threat or not, the system learns again. Soon enough, the initial pattern which cause the system to flag down the individual will be modified and a new pattern will emerge. Thus, evolving and becoming more accurate. Over time, the system performs a ‘balanced assessment of relative weights’ which is to say that a person with no criminal history acting in a suspicious manner will not be flagged down. Such as a school teacher keeping the car motor running while parked in front of a bank will not be flagged as a getaway vehicle.
It should be noted that these systems don’t pass judgement but merely calculate the propensity of an individual to commit a crime based on their recent behavior in public. And current gen PCA systems like Hitachi’s Visualization Predictive Crime Analytics simply flag down such areas which results in increased police patrolling. Such directed patrols have proven to reduce crime statistics in hot spots according to studies conducted in the USA.
Active PCA systems
As mentioned earlier, quite a few systems are already in place and have been aiding law enforcement agencies. Here are some of them.
Hitachi Visualization Predictive Crime Analytics
Hitachi’s offering uses data from the 911 dispatch system, license plate readers along the roads, gunshot sensors and other public safety systems to produce a heat map which indicates hot spots in the city with a higher probability of crime occurring. It aims to provide policing agencies with real-time insight so that these agencies can be better prepared for an incident before it even occurs – prevention is better than cure when it comes to crime, after all. It’s a hybrid cloud-based system that can be implemented on any of the popular cloud service providers.
Hitachi’s system in action
HunchLab
Made by a startup in Philadelphia by the name of Azavea, HunchLab is a platform that helps police balance resource allocation based of existing circumstances, such as the weather, crimes committed in the past few days, GPS data, etc., to generate a visualization of hotspots around a city. And by implementing tried-and-tested crime mitigation tactics, HunchLab can tell you where to deploy police officers and what situations they should expect. Accordingly, the police will be better equipped to handle the situations, and there’s less of a surprise element.
HunchLab real time Risk Scoring
IBM SPSS Crime Prediction and Prevention
IBM isn’t new to the world of AI, and rightly so, their system seems to be a lot more advanced. This platform monitors violent criminals to generate heat maps to point out which areas they frequent, and then it compares local incidents of crime with regional and national gang activities in order to extrapolate trends. These trends help the system identify criminals based on similarities related to previous crimes. Also, since it looks at previous crimes, it can identify and shortlist criminals whose modus operandi match up with observed activity. Add to this, the fact that it also looks at circumstantial data such as the weather, social events, holidays, etc. to see which circumstances make for higher probability of criminal activity.
You may never need to ask the system, instead the system might warn you…
Domain Awareness System (DAS)
Conceptualised after the 9/11 terrorism attacks. DAS is a surveillance system built by the city of New York and Microsoft to monitor the city. It obtains real-time video from over six thousand street cameras in New York and even data from radiation sensors built into patrolling boats, cars, trucks and even the gun belts that each police officer carries. The sensors are so sensitive that they are also triggered by someone who has just had radiation therapy for diseases such as cancer. The sheer amount of metadata it generates and monitors, allows the system to track down individuals with simple keywords such as “wearing a red shirt”.
The Future
Given how these systems are slowly evolving to identify trigger events which might flare up into an act of crime, one can always envision such platforms morphing into an even more perverse form. These systems are already monitoring schools so imagining a situation where children are identified early on as troublemakers would allow society to help them and mitigate crime. An even more perverse technology would result in genetic markers being observed to design babies who’ll never walk down the path of crime – if we allow it, that is.
This article was first published in October 2016 issue of Digit magazine. To read Digit's articles first, subscribe here or download the Digit e-magazine app for Android and iOS. You could also buy Digit's previous issues here.
Mithun Mohandas
Mithun Mohandas is an Indian technology journalist with 10 years of experience covering consumer technology. He is currently employed at Digit in the capacity of a Managing Editor. Mithun has a background in Computer Engineering and was an active member of the IEEE during his college days. He has a penchant for digging deep into unravelling what makes a device tick. If there's a transistor in it, Mithun's probably going to rip it apart till he finds it. At Digit, he covers processors, graphics cards, storage media, displays and networking devices aside from anything developer related. As an avid PC gamer, he prefers RTS and FPS titles, and can be quite competitive in a race to the finish line. He only gets consoles for the exclusives. He can be seen playing Valorant, World of Tanks, HITMAN and the occasional Age of Empires or being the voice behind hundreds of Digit videos. View Full Profile